YanRong Tech showcases leading performance in the latest MLPerf Storage v1.0 Benchmark, demonstrating unmatched performance, scalability, and cost-effectiveness for AI and Machine Learning infrastructures.
YanRong Tech, a pioneering leader in high-performance AI storage, announced impressive performance results in the latest MLPerf® Storage v1.0 Benchmark organized by MLCommons. These results underscore its exceptional ability to optimize AI/ML workloads and GPU performance. YanRong's all-flash storage F9000X with its high-performance distributed file system YRCloudFile, demonstrated outstanding performance and efficiency across three deep learning models: 3D-Unet, ResNet50, and CosmoFlow, taking the #1 spot in both bandwidth and the number of GPUs powered per compute node.
Storage Systems Play a Critical Role in AI Model Training Performance
New MLPerf Storage v1.0 Benchmark Results Show Storage Systems Play a Critical Role in AI Model Training Performance.
High-performance AI training, particularly for large language models (LLMs), demands storage systems that are both scalable and high-speed. Without this, data access quickly becomes a bottleneck, throttling overall system performance. As highlighted in a recent study, data management (35%) poses a more significant technological challenge than computing power (26%) when scaling AI models. This is often due to outdated data architectures and insufficient I/O capabilities within organizations.
As accelerator technologies like GPUs advance, and as datasets grow larger, storage solutions must evolve to keep pace with compute demands. Maximizing data throughput is crucial for ensuring optimal GPU utilization, which accelerates model training, improves accuracy, and reduces operational costs.
As enterprises increasingly adopt AI, storage systems must be able to support a wide range of AI tasks. Ensuring infrastructure can scale and deliver data at the speeds required by modern AI workloads is critical to achieving time-to-business value and successfully deploying AI applications.
Imagine a solution that meets all your AI workload demands. YanRong's high-performance distributed file system, YRCloudFile, offers the perfect answer, delivering robust storage and a unified experience to run your AI applications efficiently across various environments.
MLPerf Storage Benchmark and Its Impact
The MLPerf Storage benchmark, an internationally recognized AI performance benchmark, is the first and only open, transparent benchmark to measure storage performance in a diverse set of ML training scenarios. It simulates the storage demands across diverse models, accelerators, and workloads without needing actual accelerators by emulating their "think time". The benchmark focuses the test on a given storage system's ability to keep pace, as it requires simulated accelerators to maintain a required level of utilization.
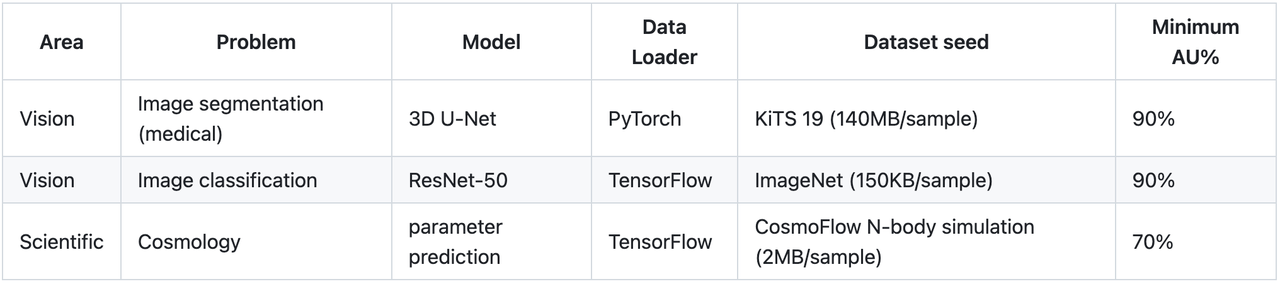
The v1.0 benchmark emulates NVIDIA A100 and H100 AI accelerator models as representatives of the current generation of accelerator technology. It tests storage system performance in three AI models—3D-Unet, ResNet50, and CosmoFlow—covering a wide range of sample sizes, ranging from hundreds of megabytes to hundreds of kilobytes, as well as wide-ranging simulated “think times” from a few milliseconds to a few hundred milliseconds.
MLPerf Storage benchmark offers a standardized method for vendors to measure storage performance, helping end-users estimate storage needs. In addition, based on storage capabilities, it will allow users to estimate the optimal amount of accelerators for their particular environment.
YanRong Sets New MLPerf Standards in AI/ML Storage Performance
YanRong Tech participated in the MLPerf test using its newly released all-flash storage product, F9000X. Each storage node of the F9000X is equipped with the latest Intel® Xeon® 5th Generation Scalable Processors, utilizing 10 Memblaze PCIe 5.0 NVMe SSDs, along with 2 NVIDIA ConnectX-7 400Gb NDR network cards. The network topology of the test environment is shown in the figure.
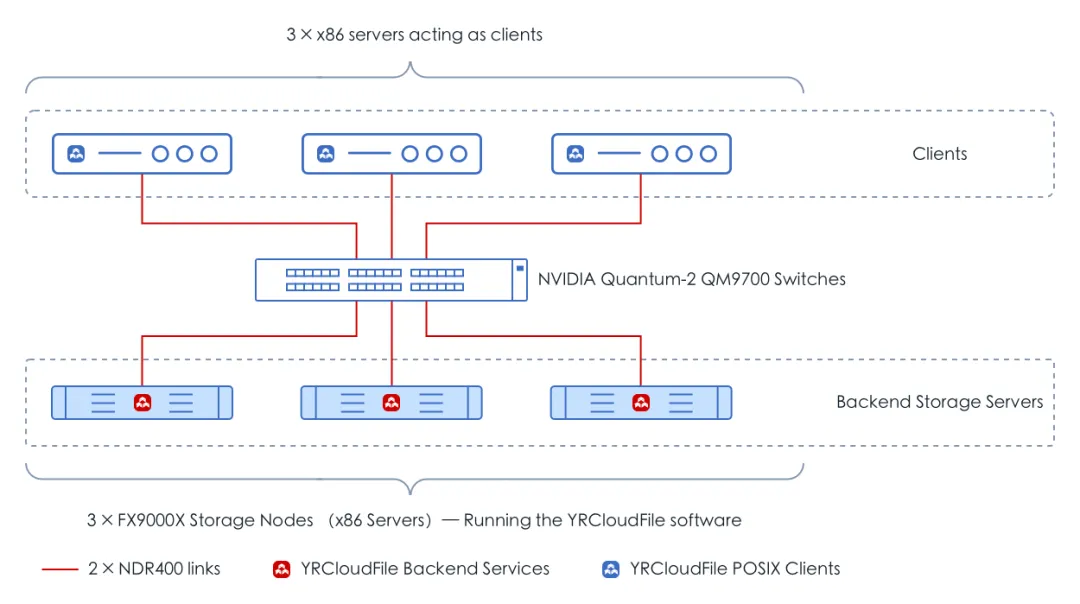
MLPerf Benchmark Achievements include:
On a single host, YanRong F9000X can sustain up to:
- 60x H100 accelerators training CosmoFlow at 34 GB/s
- 188x H100 accelerators training ResNet50 at 37 GB/s
- 20x H100 accelerators training UNet3D at 58 GB/s
In tests with 3 hosts, YanRong F9000X can sustain up to:
- 120x H100 accelerators training CosmoFlow at 72 GB/s
- 540x H100 accelerators training ResNet50 at 103 GB/s
- 60x H100 accelerators training UNet3D at 169 GB/s
The only storage vendor in China that participated in all three model tests
YanRong Tech is the only storage vendor in China that participated in all AI model tests of the MLPerf Storage v1.0 benchmark, which includes 3D-Unet, CosmoFlow, and ResNet50. And YanRong F9000X all-flash storage demonstrated exceptional performance, fully addressing the data workload requirements of currently mainstream model applications. It is capable of handling large-scale datasets and can elastically scale according to the size of the AI cluster, perfectly matching GPU compute demands.
#1 in both bandwidth and the number of GPUs powered per compute node
The MLPerf Storage benchmark rules define that a single compute node (client) can run multiple accelerators (ACCs in short) for the corresponding model application tests, while also supporting large-scale distributed training cluster scenarios. Multiple clients simulate concurrent access to the storage cluster in a manner that reflects real data processing. The greater the average number of ACCs that each client can run, the stronger the compute capability of that node, allowing it to handle more tasks, which in turn increases the requirements for concurrent access performance to the storage system.
As shown in the figures below, in the distributed training cluster scenario, YanRong Tech ranked first in both the average number of ACCs and storage bandwidth performance supported per compute node across all three model tests, clearly establishing its unquestionable leadership position in AI storage.
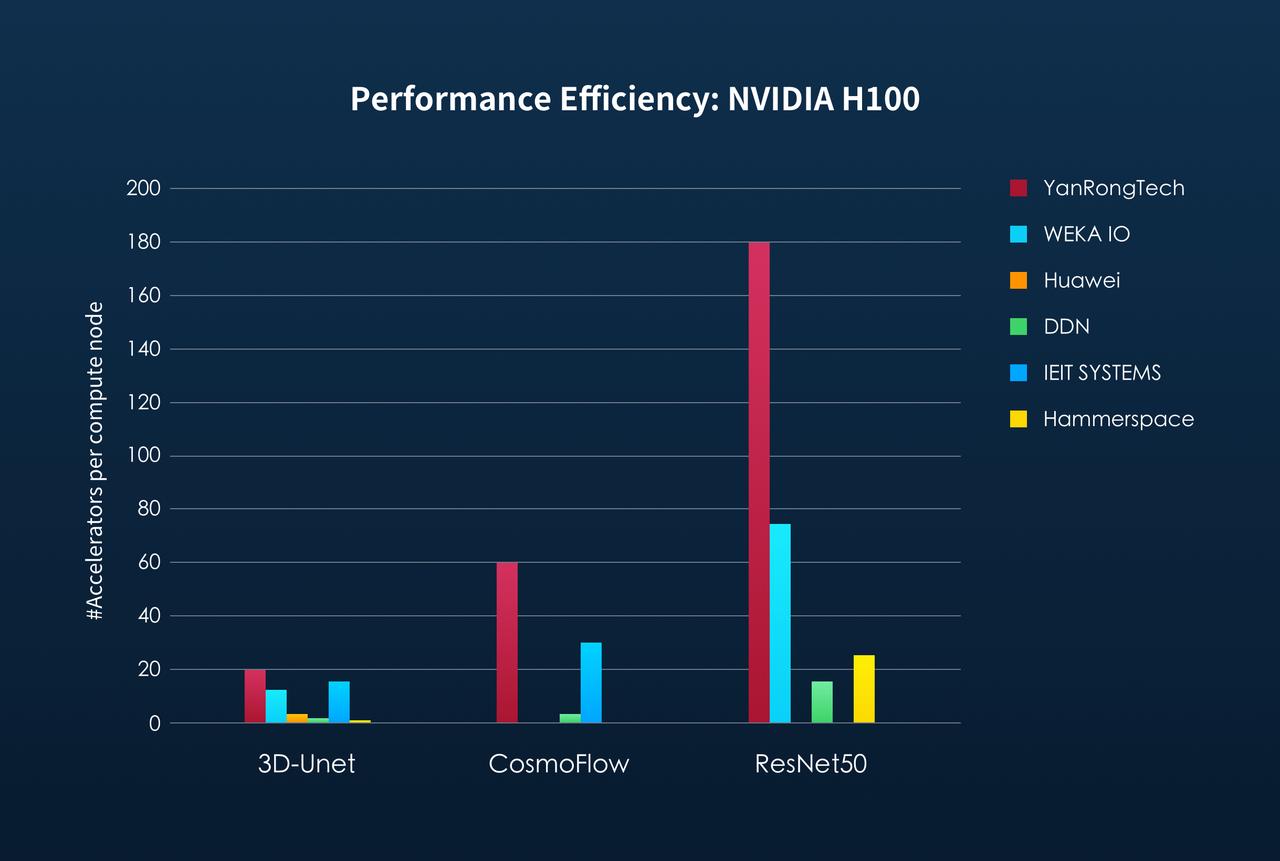

Storage performance increases linearly with computational scale
As the scale of computation expands, storage performance should achieve linear growth to meet the demands of AI training. Taking the three-dimensional image segmentation model,3D-Unet, as an example, the size of a single image sample is approximately 146MB. In a multi-node cluster environment, the number of training samples processed per second can exceed 1,100, resulting in a bandwidth requirement for reading training data that exceeds 160GB/s.
Across all three tested models, YanRong's all-flash storage F9000X exhibited exceptional linear scaling. The benchmark results showed that as the number of concurrent compute nodes (ACCs) increased, the storage system's bandwidth performance maintained a significant linear growth capability. Furthermore, the Accelerator Utilization (AU) consistently remained within the range required by MLPerf benchmark, ensuring the efficiency and stability of the AI training process.
In the MLPerf benchmark of the 3D-Unet model, using three compute nodes, YanRong F9000X can simulate 60 H100 ACCs, achieving a bandwidth of 160GB/s. The F9000X three-node storage cluster has been tested to reach a maximum bandwidth performance of over 260GB/s, indicating that in practical business environments, YanRong's all-flash storage can support more GPU compute nodes.
The following three figures show the storage availability (AU) and bandwidth performance of YanRong's all-flash storage in the 3D-Unet, ResNet50 and CosmoFlow models:
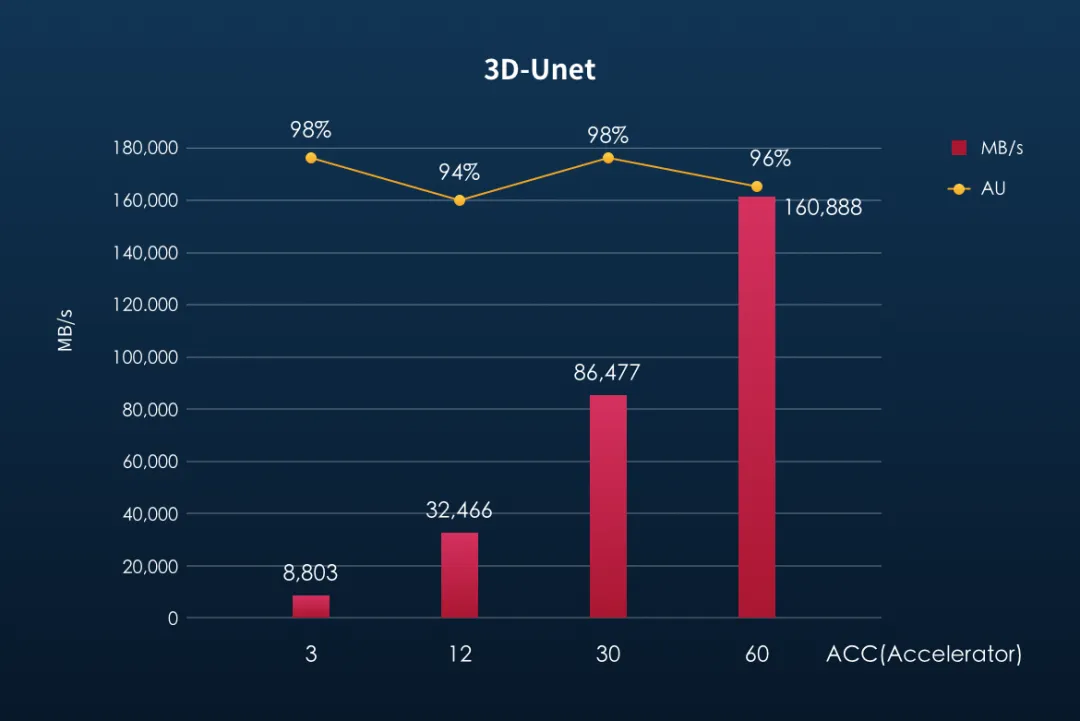
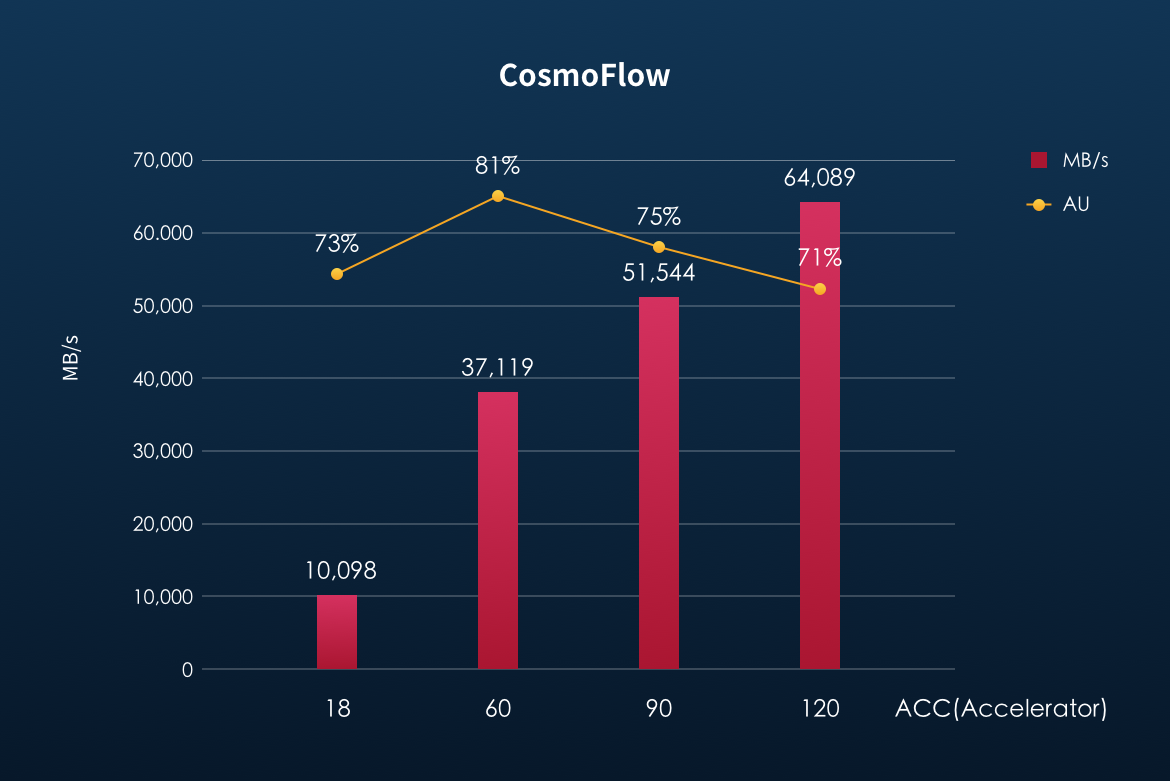
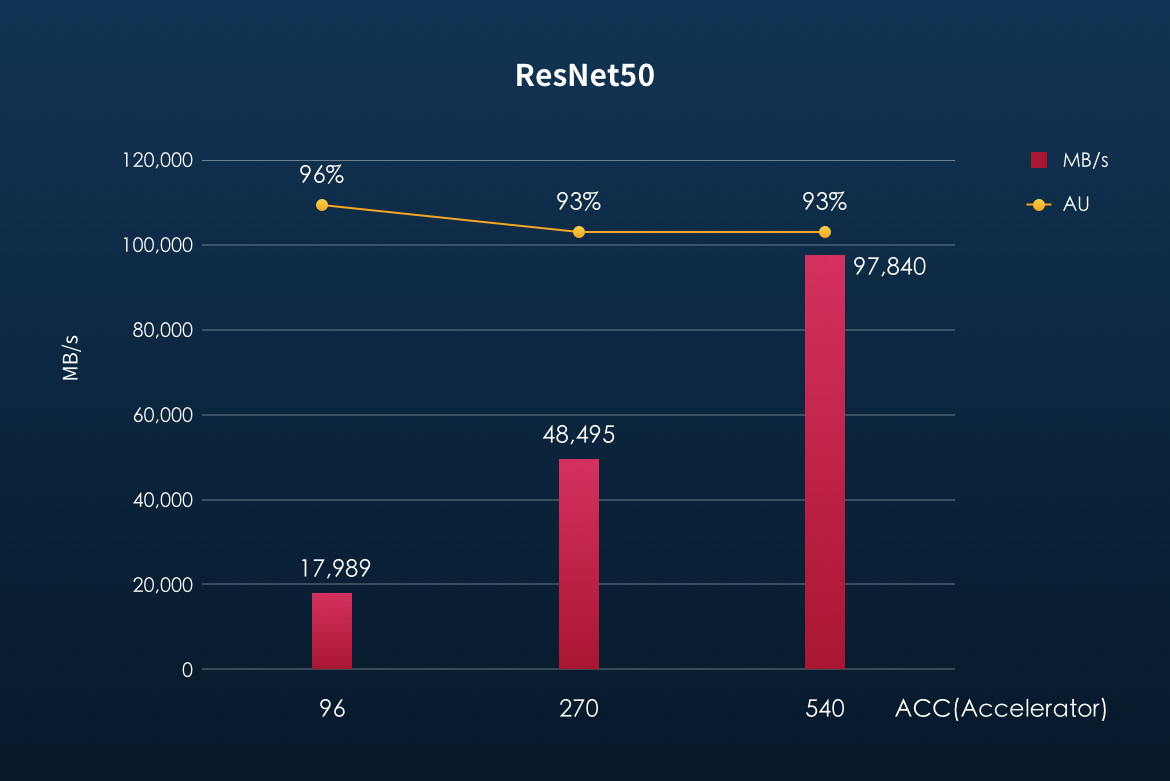
The AI/ML-Ready Storage
That's been the goal of YanRong since day one: help our customers access unparalleled performance and economic success through highly advanced storage tailored specifically to hybrid cloud and AI. The MLPerf benchmark results validates the strength of our current offering and vision, highlighting our commitment to pushing the boundaries of high-performance, high-throughput and scalable file storage solutions for AI and machine learning.
As AI and machine learning workloads are poised to grow exponentially, efficient GPU utilization and fast access to training data are critical. YanRong is working hard on further improvements for the future, ensuring that storage bottlenecks are eliminated, enabling organizations to fully leverage their GPU resources and accelerate model training and inference times even further.
About YanRong
YanRong is a high performance storage leader in AI and HPC at scale. YRCloudFile is a high-performance, data center-level distributed shared file system product built specifically for software-defined environments, providing customers with powerful storage to help them better manage and utilize unstructured data assets. Users can easily deploy YRCloudFile to build a fast, highly scalable and resilient file system for their AI and high-performance workloads.